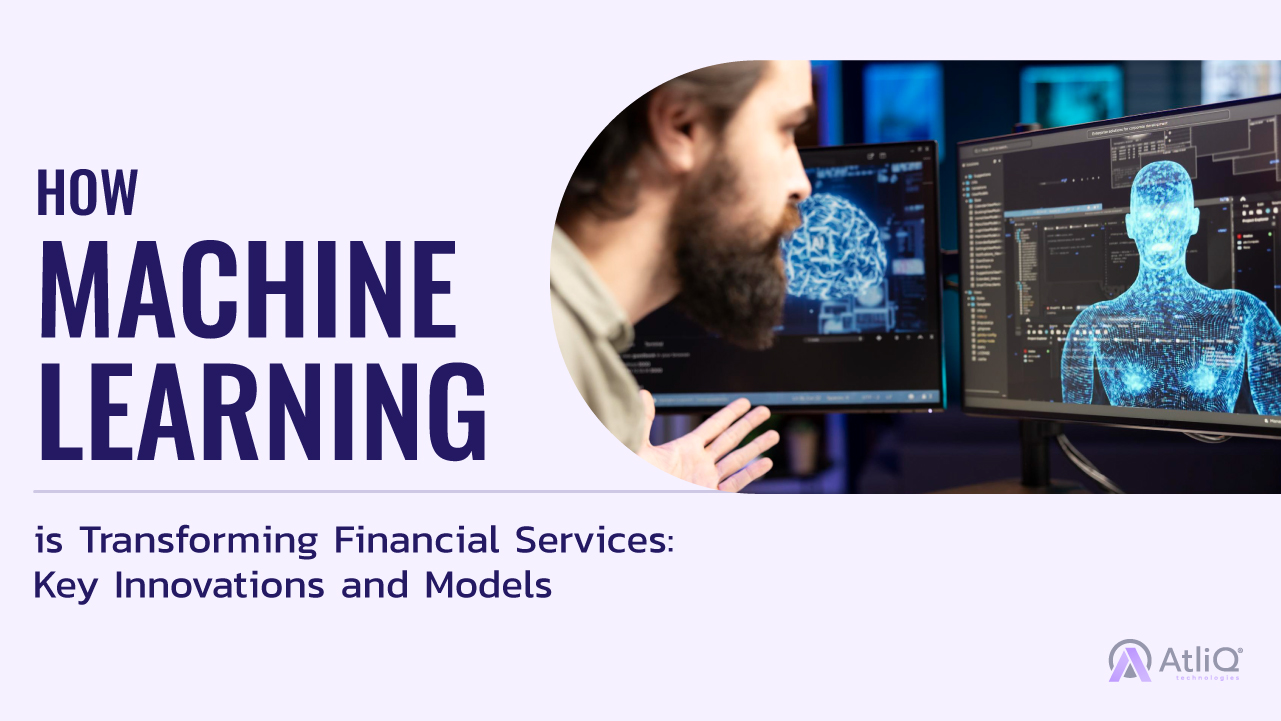
In the rapidly evolving world of financial services, innovation isn’t just an option—it’s a necessity. Machine Learning is at the heart of this transformation, reshaping how financial institutions operate and deliver value.
According to a recent report by McKinsey, 73% of financial services firms are investing in AI and ML technologies to stay ahead of the curve. From detecting fraud in milliseconds to personalizing customer experiences with pinpoint accuracy, ML is not just enhancing existing systems but revolutionizing them. Imagine a future where your bank’s next best investment opportunity is identified before you even ask.
This isn’t science fiction; it’s the power of ML in action.
Definition Machine Learning
ML is a subset of artificial intelligence that enables systems to learn and improve from experience without being explicitly programmed. At its core, ML involves algorithms that process data, recognize patterns, and make decisions or predictions based on those patterns. These algorithms can range from simple linear models to complex neural networks, each suited to different types of data and applications.
How ML Transforms Traditional Financial Services
Traditionally, financial services relied heavily on manual processes and static models to make decisions, whether it was assessing loan applications or detecting fraudulent activities. ML revolutionizes this landscape by introducing dynamic, data-driven approaches that continuously evolve. Instead of static rules, ML models adapt and refine their predictions based on new data, leading to more accurate and timely insights.
For instance, where traditional credit scoring might rely on a set of predefined criteria, ML models analyze a broader spectrum of data, including transaction history and behavioral patterns, to assess creditworthiness more comprehensively. This ability to process vast amounts of data and adapt in real time is transforming how financial services operate.
Key Innovations in ML Model Development
Algorithmic Trading: Algorithmic trading refers to the use of computer algorithms to execute trading orders at speeds and frequencies far beyond human capabilities. ML models play a crucial role in this space by analyzing vast amounts of market data, identifying patterns, and making split-second trading decisions. These models can adapt to changing market conditions and refine their strategies based on new data.
For example, deep learning algorithms can process historical market data and real-time information to forecast price movements and optimize trading strategies. An ensemble model might combine predictions from multiple algorithms to enhance accuracy and reduce risk. The benefits of algorithmic trading include increased execution speed, reduced human error, and the ability to leverage complex trading strategies that would be impossible to implement manually.
Credit Scoring and Risk Assessment: Machine learning enhances traditional credit scoring models by incorporating a broader range of data sources and more sophisticated analytical techniques. Traditional credit scores often rely on limited financial history, which can be inadequate for accurately assessing creditworthiness. ML models, however, can analyze diverse data, including transaction history, spending behavior, and even social media activity, to provide a more nuanced view of a borrower’s risk profile.
Predictive risk assessment using ML offers several benefits, such as improved accuracy in identifying high-risk borrowers and better prediction of default probabilities. For example, a gradient boosting model might integrate various features to predict loan default more precisely than traditional models. This results in more informed lending decisions and reduced risk for financial institutions.
Fraud Detection: In the realm of fraud detection, ML models are instrumental in identifying and preventing fraudulent activities by analyzing transaction patterns and detecting anomalies. Unlike traditional rule-based systems, ML models can continuously learn from new data and adapt to emerging fraud patterns.
For instance, a supervised learning model can be trained on historical fraud data to recognize patterns associated with fraudulent transactions. Case studies, such as the implementation of ML systems by major banks, have shown significant reductions in fraud rates. One notable example is the use of neural networks by a financial institution to identify unusual transaction patterns, resulting in a 30% decrease in fraud cases within the first year.
Customer Personalization: Machine learning drives personalized financial services by analyzing individual customer data to provide tailored advice and recommendations. ML models can assess a customer’s financial situation, preferences, and goals to offer personalized investment strategies, product recommendations, and financial planning advice.
For example, a collaborative filtering model used by fintech companies can analyze customer behavior and preferences to suggest investment opportunities or financial products that align with their needs. Another example is recommendation engines that personalize marketing offers based on a user’s transaction history and behavior. These personalized services not only enhance customer satisfaction but also increase engagement and loyalty.
Challenges in ML Model Development for Financial Services
Data Privacy and Security: Protecting sensitive financial data is crucial. Implement encryption, secure access, and techniques like differential privacy to ensure data confidentiality. Stay vigilant against breaches and update security practices regularly.
Model Accuracy and Bias: Accuracy is key for reliable decisions. Use diverse datasets and techniques like cross-validation to detect and correct biases. Regularly test and refine models to ensure fairness and precision.
Regulatory Compliance: Compliance with regulations such as GDPR is essential. Ensure transparency in model decisions, maintain thorough documentation, and stay updated on regulatory changes. Collaborate with legal teams to meet all requirements.
Future Trends in ML for Financial Services
Emerging Trends and Technologies: The future of ML in financial services is bright with emerging technologies like quantum computing and AI-driven fintech. Quantum computing promises to enhance computational power, enabling more complex and efficient financial modeling. AI-driven fintech is revolutionizing how services are delivered, offering more personalized and automated solutions.
Predictions for Future Impact: ML is set to further transform financial services by enhancing predictive accuracy, optimizing decision-making, and automating complex processes. Expect more sophisticated risk management, improved fraud detection, and highly personalized customer experiences. As these technologies evolve, they will drive innovation, efficiency, and competitive advantage in the industry.
Machine Learning is undeniably reshaping the financial services landscape, driving innovations that enhance efficiency, accuracy, and personalization. From sophisticated fraud detection to dynamic trading strategies and improved credit scoring, ML is at the forefront of transforming how financial institutions operate. As technologies like quantum computing and AI-driven fintech emerge, the potential for ML to further revolutionize the industry is immense. Staying ahead of these trends will be key to leveraging ML’s full potential and maintaining a competitive edge.